Marketing Mix Modeling for Atypical Brands: Can It Work for You?

Marketing Mix Modeling (MMM) is a widely used technique to measure the impact of paid advertising on sales. To those new to MMM, it might feel complex and even hard to believe but it is really just the application of econometric statistical techniques to an advertising or marketing question. A major part of econometrics is concerned with “time series questions”, where one has a time series of data measured at regular increments for some extended period of time – let's say interest rates and unemployment. An economist examines how interest rates and the unemployment rate vary over time, rather than basing their analysis on the current rate of each. Do they both go up/down together, do changes in interest rates lead to future changes in unemployment? What is the magnitude of the interactions?
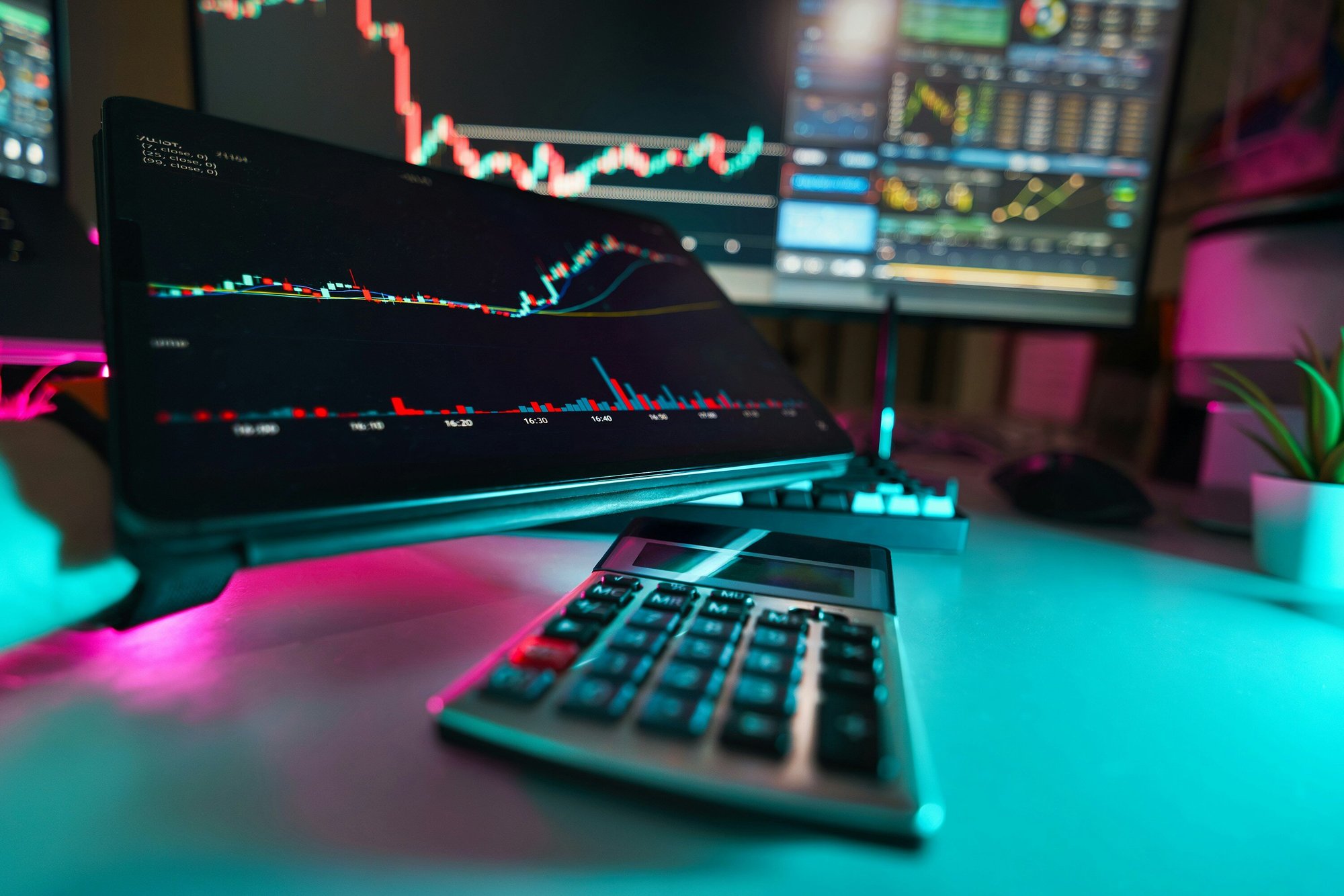
That’s really all we’re doing for Marketing Mix Modeling: analyzing how changes in media over time relate to fluctuations in sales.
That sounds straight forward for many industries: I sell cheese every day, and I run media every day (ok, at least most days). Lots of other non-media factors also change such as price, promotions, what’s on shelf at the store, but I also have data on this. So now I can look at how all these things have changed, week to week or day to day, over the past few years. I can even do this for each market or each retailer, so now I have lots (and lots) of variation over time.
But not every business has this luxury. Some brands only sell their product for a few months a year. Other brands don’t even use paid media to directly drive “a sale”.
Over the years, we’ve helped many brands with a less traditional “time series” find ways to effectively apply marketing mix modeling to quantify the impact of media and optimize budget allocations to save money and drive growth.
“Selling Season” Brands
Now we don’t mean brands with a seasonal sales pattern: boxes of stuffing mix hit peak sales each Thanksgiving, Hot Dogs peak each 4th of July holiday, and many clothing retailers hit their peak each December. That’s just normal seasonality and has never really been a problem or a challenge. In fact, that sort of seasonality is even a consideration for the economists we spoke of earlier. When we refer to “selling season” brands we mean brands that sell ONLY for a brief period each year. Now you might think of movie or theater tickets, that’s slightly different because they essentially have a new brand each sales wave. We mean lasting brands that consumers have known and trusted for decades but only have a brief window each year to sell. Brands like tax preparation services that only have customers from December to April, or Baseball teams that only sell tickets leading up to and during their season.
To the non-statistician this situation may not seem so alarming; we still have a time series, it’s just that for many weeks/days the values are all zero! The problem is that without proper thinking – aka analytics exploration – the standard statistical techniques are going to struggle to separate this extreme seasonality from media impact. In season there are always both sales and media; out of season there is always zero media and zero sales – so what’s the baseline? What would you sell without any media spend?
While every brand has unique aspects, the key to modeling “selling season” brands is generally to find base drivers (non-marketing factors that impact sales) that sit in between pure seasonality and media. We find that many brands have these metrics, but only when one digs in and connects with the right folks.
Creating the proper base controls requires data exploration and thinking about the situation from the consumer’s point of view. The Media team is rarely enough. When the business doesn’t fit neatly into the time series measurement box the solution to discovering base drivers often comes from job functions outside of media – and sometimes outside of marketing altogether. To achieve success, we require individuals with extensive experience in MMM and a toolkit that facilitates practical, timely, and transparent testing. These engagements can never be fully specified in advance because testing hypothesis is core to the task, so the reality is that the MMM partners must be nimble while staying on budget and within timelines.
For example, consider how a consumer might see baseball tickets for sale. On any given day during the season, the baseball fan can purchase tickets to a game today, or any game in the future. We would expect ticket sales to increase when there is a home game today or the next week, and do the games feature key rivals. Also, there are more potential games available early in the season vs. later. These items can be explored and tested to create a good base model, before testing the media drivers.
When the KPI is not a sale
Another case for atypical marketing mix modeling is when the true role of media is to drive something that leads to a sale but is not a literal sale (i.e. product sale, subscription, etc.).
The broader healthcare industry is full of these examples. Health Care Providers have a complex payment system intertwined with insurance policies that vary by patient and location. Marketing’s role is to lead patients to choose the target brand vs. other available health systems so the KPI for marketing mix modeling needs to be closer to the patient decision – it should be something like appointments being booked or encounters with patients. The choice should ultimately be made by carefully understanding the provider’s business and the intended outcome of the media.
One might assume that any given business has one challenge: to acquire and maintain customers, but sometimes this can be multifaceted. Some brands must first win the right to sell to a specific audience group and then convince members of the group to buy. We see this with some part of the health insurance industry. They must simultaneously run B2B media to win the right to sell and B2C media to convert individuals once they are eligible. In this situation they need two KPIs, but the consumer/patient sale is dependent on the B2B win. Marketing Mix Modeling is still possible, but with this sort of complexity it is even more important that the MMM team fully comprehend the situation and that the MMM tools are efficient and robust enough to test an added level of complexity.
Conclusion
For a successful MMM, reliable marketing data is necessary but not always sufficient – especially within non-standard industries. When data is challenging, creative thinking and exploration can enable almost any business to benefit from MMM.
So, key takeaways:
- Base drivers are important and benefit the MMM by better isolating the media impacts from other sales drivers
- Creating the proper base controls requires data exploration - thinking about the situation from the consumers point of view and testing within models
- Your broad organization probably knows more about base drivers than you may think
- It is worth the effort to create base metrics that are customized to your business (i.e. turn a known phenomenon into a data series)
Ironically, measuring media effectiveness often depends on understanding everything except media. With the right mix of expertise, flexibility, and data-driven creativity, even atypical brands can harness the power of MMM to drive smarter decisions.